Water Utilities Call on Big Data to Guide Pipe Replacements
The drinking water industry says aging infrastructure is its top challenge. Can AI help?
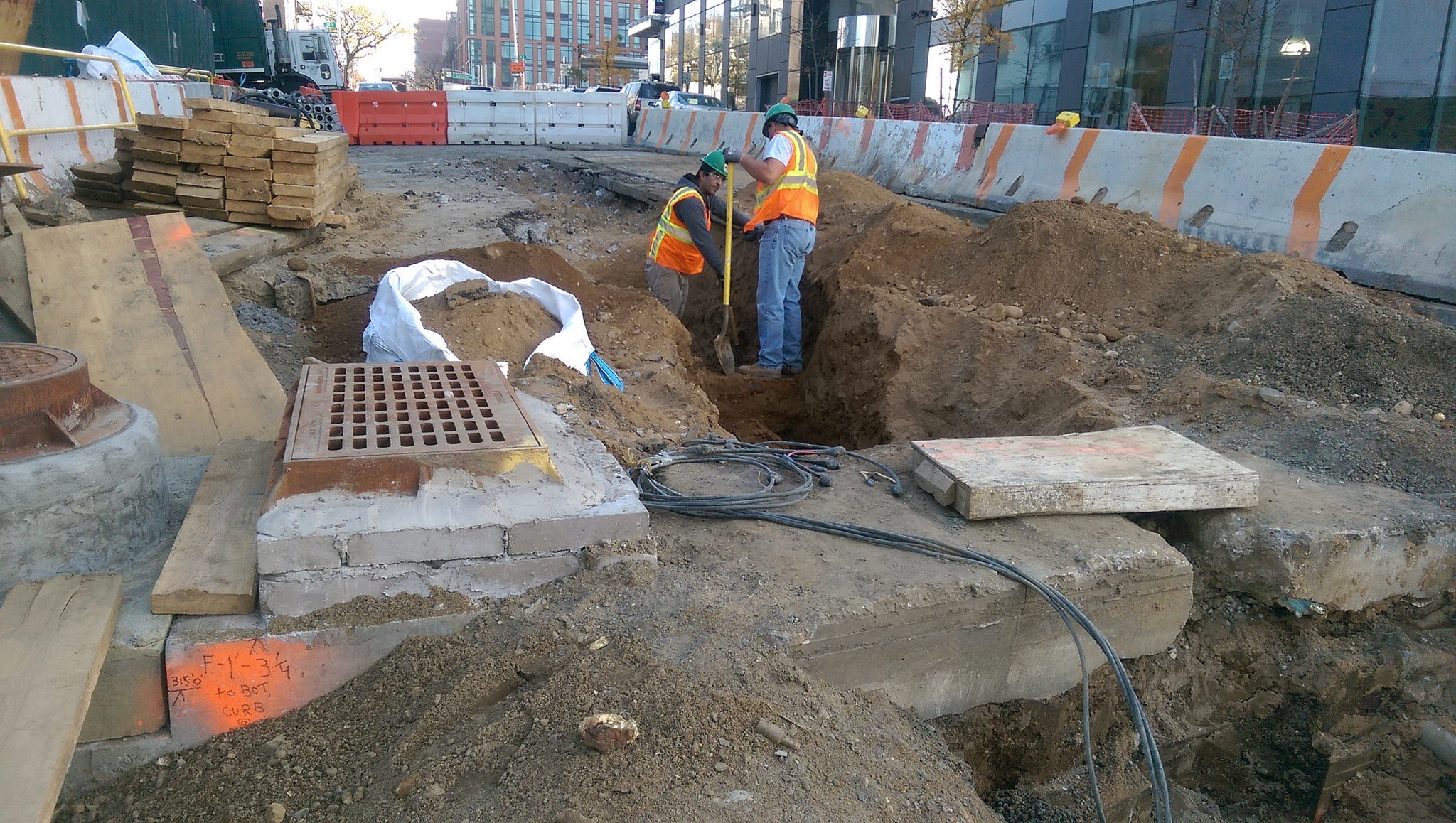
Workers in Manhattan excavate 23rd Street to replace the water main. Photo courtesy of Flickr/Creative Commons user MTA
By Brett Walton, Circle of Blue
- For five consecutive years, water professionals surveyed by the American Water Works Association have ranked replacement of aging infrastructure as the industry’s top challenge
- Artificial intelligence and machine learning, combined with utility pipe-break archives and data about environmental factors like flow rates, soil chemistry, and temperature can pinpoint which pipes are most likely to fail and thus are the best candidates for replacement
- Only one out of five utilities surveyed last year by the American Water Works Association said that they use data mining to improve the operation and maintenance of their water and sewer systems
Doing surgery on San Francisco’s water system is no simple task. Replacing one mile of distribution main costs about $3.8 million dollars. That’s just the direct cost of installing a section of drinking water pipe. There are also side effects: disruptions to traffic, sidewalks, and businesses when streets are pried open.
In one of the nation’s densest and highest-cost cities the expense amounts to an incentive for well-informed decisions about what to dig up and when.
“To leave good pipe in the ground as long as possible is economically important,” said Katie Miller, manager of the water distribution division at San Francisco Public Utilities Commission.
But, out of the city’s more than 1,200 miles of water mains, which are the good ones? Age is a clue but not a conclusion. Miller told Circle of Blue that some pipes beneath San Francisco that were installed more than a century ago are still in adequate condition, whereas other pipes half as old, but made from inferior materials, are cracking.
To leave good pipe in the ground as long as possible is economically important.” — Katie Miller, San Francisco PUC
Detailed data can help separate the good from the bad, and San Francisco PUC and a handful of other utilities are turning to a growing cluster of analytics companies to help them make sense of it. The hope is that the tools of artificial intelligence and machine learning, combined with utility pipe-break archives and data about environmental factors like flow rates, soil chemistry, and temperature can pinpoint which pipes are most likely to fail and thus are the best candidates for replacement.
The need for precision has never been greater. For five consecutive years, water professionals surveyed by the American Water Works Association have ranked replacement of aging infrastructure as the industry’s top challenge, above long-term water availability, cybersecurity, watershed protection, financing, and other pressures. The trade organization also estimates that the replacement tab for America’s water pipes will run close to $1 trillion over 25 years. Meanwhile, more water mains are breaking and maintenance costs are growing at an increasing pace, taking up a larger share of utility budgets. With water rates already rising and the affordability of drinking water service for the poor an emerging public policy concern, utilities have reason to be selective in their pipe replacement programs.
“It’s about using ratepayer money most effectively,” said David Katzev of East Bay Municipal Utility District, which is using AI tools. Repairing a broken pipe costs between $10,000 and $15,000, he estimated. Replacement is several orders of magnitude more expensive, roughly $2.5 million per mile in East Bay MUD’s service area on the east side of San Francisco Bay. “It’s so costly to replace pipe that we want to make sure that we’re replacing the right one.”
From Reaction to Prediction
Digital technologies and Big Data — using computing power to find patterns in large sets of numbers — are transforming, albeit slowly, even the most hidebound industries, including municipal water utilities. Sensors detect changes in sewer systems flows in real-time, alerting managers to the possibility of an overflow. Smart meters track household water use by the hour, allowing leaks to be noticed more quickly. Bluefield Research, which follows water utility trends, says that using data insights to improve the performance of their assets could save utilities billions of dollars in the next decade. Industry heavyweights and startups alike are angling for a piece of the data analytics market.
Both East Bay MUD and San Francisco PUC are working with a company called Fracta to help guide their pipe replacement decisions. Both utilities are in the early stages of increasing annual water main replacement. East Bay MUD replaced 15 miles of water mains last year, and plans to replace 20 miles a year by 2020. The long-term target is 40 miles per year. With data analysis, the aim is to move from reaction to prediction, to “get ahead of the game,” said Katzev, a civil engineer who is managing the utility’s pipeline rebuild program.
A subsidiary of Kurita Water Technologies, a Japanese firm, Fracta started life with physical assessments. The company, when it was operating under the name HiBot USA, sent robots into pipes to scope out the interior walls, mostly in oil and gas lines.
Physical assessments turned out to be too slow and expensive so company leaders turned to number crunching instead. They read the American Water Works Association pipe replacement report and saw an opportunity in the water sector.
The Fracta recipe takes utility data on pipe age, material, and break history and adds in dozens of additional environmental factors like soil chemistry, soil slopes, air and water temperature, and proximity to other infrastructure. Those data streams, after being cleaned for missing or incomplete numbers, are fed to computer algorithms that look for correlations between data points. How do ductile iron pipes in low pH soils in the city center compare with an asbestos cement pipe that crosses beneath a freeway in a low-lying area?
“Machine learning looks at all of that and identifies that these variables in this setting are associated with a break with this type of pipe,” said Doug Hatler of Fracta. The more data to feed the model, the better it performs, he said.
After running the computer model, Fracta then gives each section of pipe a risk score, from low- to high-probability of a break.
So far the experiment is proving fruitful for San Francisco PUC and East Bay MUD.
“Our breaks are all happening in high-risk-of-failure pipes,” Miller said. Her team, which started working with Fracta in 2016, is now using the risk scores to deploy acoustic sensors that listen for leaks in the pipes most likely to fail.
Katzev also says that early results look promising.
Success will be measured in a long-term decline in the utility’s water main break rate, he said. But it’s too soon for those conclusions.
“I don’t think one or two years is going to get us there,” Katzev said.
A Tool, Not Magic
Big Data has promise — “The marriage of data and water lay the foundation for tomorrow’s smarter cities,” proclaimed one industry publication last year — but for now it’s more buzz than honey for most water utilities.
Like a child, AI can learn bad things if the data is not good.” — Sunil Sinha, Virginia Tech
Only one out of five utilities surveyed last year by the American Water Works Association said that they use data mining to improve the operation and maintenance of their water and sewer systems. Water officials, according to the report, “remain unconvinced” about the long-term potential of the data revolution that is remaking other industries.
“It’s definitely still early days,” said Will Maize, a research director with Bluefield Research. Maize told Circle of Blue that East Bay MUD is known within the industry as an early adopter, willing to sample new technologies. Because it does not involve construction or integrating new hardware, the predictive tool offered by Fracta is even less of a leap. “It’s a really low-risk opportunity for these utilities,” he said.
But what works for East Bay MUD and San Francisco PUC might not apply elsewhere.
Sunil Sinha, an engineering professor at Virginia Tech, thinks that talk of a Big Data revolution for predicting water pipe breaks is premature. Utility data is generally not detailed enough for computer modeling to have an advantage over traditional statistical methods, he said.
“Like a child, AI can learn bad things if the data is not good,” Sinha said.
And Sinha and colleagues are finding bad data everywhere they look. They are heading up a national evaluation of water distribution pipes. Called PIPEiD, the project will collect data from 500 utilities to produce a pipe performance database that utilities can use to gauge the health of their own systems.
Part of the project is determining which data are most crucial. “The majority of utilities do not collect good data,” Sinha said. Most simply record a break as a break, not marking whether it was across the length of the pipe, around its circumference, or a spot of corrosion. Nor do they track physical data that affects pipe health: water pressure, for example, or water and soil chemistry.
Maize agreed that data is an obstacle. “Data quality is certainly one thing that advanced asset players run up against,” he said.
Though San Francisco PUC generally has high quality data, it wasn’t until recently that it was complete, consistent, and accessible, Miller said. The utility has digitized four decades of its pipe break data, which stretches across 100 years. When extracting data from the old plumber’s logs, staff found that the records were mostly complete, but they found a number of errors that had to be reconciled.
“The break might have been recorded as being on a six-inch pipe, but the pipe in the ground is sixteen inches,” Miller said, outlining a hypothetical scenario. “Did they record the street wrong or get the pipe length wrong?” Reconciling these inconsistencies and digitizing the files is expensive and time-consuming, she said. But it’s necessary for Big Data methods.
Katzev said that East Bay MUD has main break data since 1990. Prior records have disappeared. “It would be nice to have earlier main break data,” he said. “It was on paper, but we just lost it.”
Sinha, whose doctoral work was in artificial intelligence, repeatedly leans on medical metaphors — “Just because a baby is born, it doesn’t mean it will live 80 to 90 years. Same with pipes.” — to describe water systems and AI networks.
Tracking breaks is like tracking heart attacks, he said. The data can identify a pattern, but it is not going to stop heart attacks. The long-term challenge is changing the behaviors that resulted in the emergency. For utilities that means taking better care of their pipes, a task that is easier for those, like San Francisco PUC and East Bay MUD, that have the funds, expertise, and management.
One small West Virginia utility that Sinha is working with on the PIPEiD project is particularly stricken, he said. Most of its budget, managers told him, is going to pipe repairs.
“They’re running the business like a hospital,” Sinha commented. “They’re in crisis mode all the time.”
Brett writes about agriculture, energy, infrastructure, and the politics and economics of water in the United States. He also writes the Federal Water Tap, Circle of Blue’s weekly digest of U.S. government water news. He is the winner of two Society of Environmental Journalists reporting awards, one of the top honors in American environmental journalism: first place for explanatory reporting for a series on septic system pollution in the United States(2016) and third place for beat reporting in a small market (2014). He received the Sierra Club’s Distinguished Service Award in 2018. Brett lives in Seattle, where he hikes the mountains and bakes pies. Contact Brett Walton
Leave a Reply
Want to join the discussion?Feel free to contribute!